In the past year, enthusiasm for the potential offered by AI agents has captured our collective imagination. How much more efficient could the average worker be with an autonomous AI assistant taking care of everything from maintaining their calendar to providing on-demand reports and analyses from databases and CRMs? The amount of time and cost savings is enough to inspire leaders in every industry.
But the journey to successfully deploying an AI agent in the enterprise is long and challenging. Many of the challenges present in launching successful generative AI applications and AI systems will be amplified with agentic AI — and 80% of generative AI projects still fail. In this blog post, we’ll explore how leaders can build an effective and adaptable strategy for agentic AI.
What is agentic AI?
Agentic AI refers to artificial intelligence systems that can autonomously or semi-autonomously take actions to achieve specific goals on behalf of users or organizations. We can think of it as the natural next step from generative AI. Generative AI systems, such as ChatGPT, enable people to make things more efficiently — whether it’s new text, image, or video content. AI-powered agents enable people to do things more efficiently — from making a dinner reservation or sending an email to performing complex data extraction tasks.
Where GenAI systems can provide information to users, AI agents can make data-backed recommendations and use real-world tools like APIs, applications, and databases to execute specific, complex tasks. AI agents are:
- Goal oriented
- Capable of action and problem solving
- Adaptable to their environment and case-by-case context
- Integrated with both people and various tools and databases
- Proactive and autonomous
- Teachable with human supervision and feedback loops
While agentic AI presents enormous opportunity, they have plenty of limits, especially as they exist today. AI agents are not “runaway AI” or artificial general intelligence — they will require human oversight and input to work.When exploring options for an agentic AI project, enterprise leaders will want to keep in mind that not all problems can (or should) be tackled with an AI agent. Be sure to avoid the following tasks and use cases:
- Providing information like traditional AI chatbots: This is a generative AI use case, as the AI output is information, rather than specific tasks.
- Completing static or scripted tasks: These can be tackled by an automated workflow or process instead.
- Working completely unsupervised: AI agents will require some level of human intervention and input to function successfully.
How AI agents work
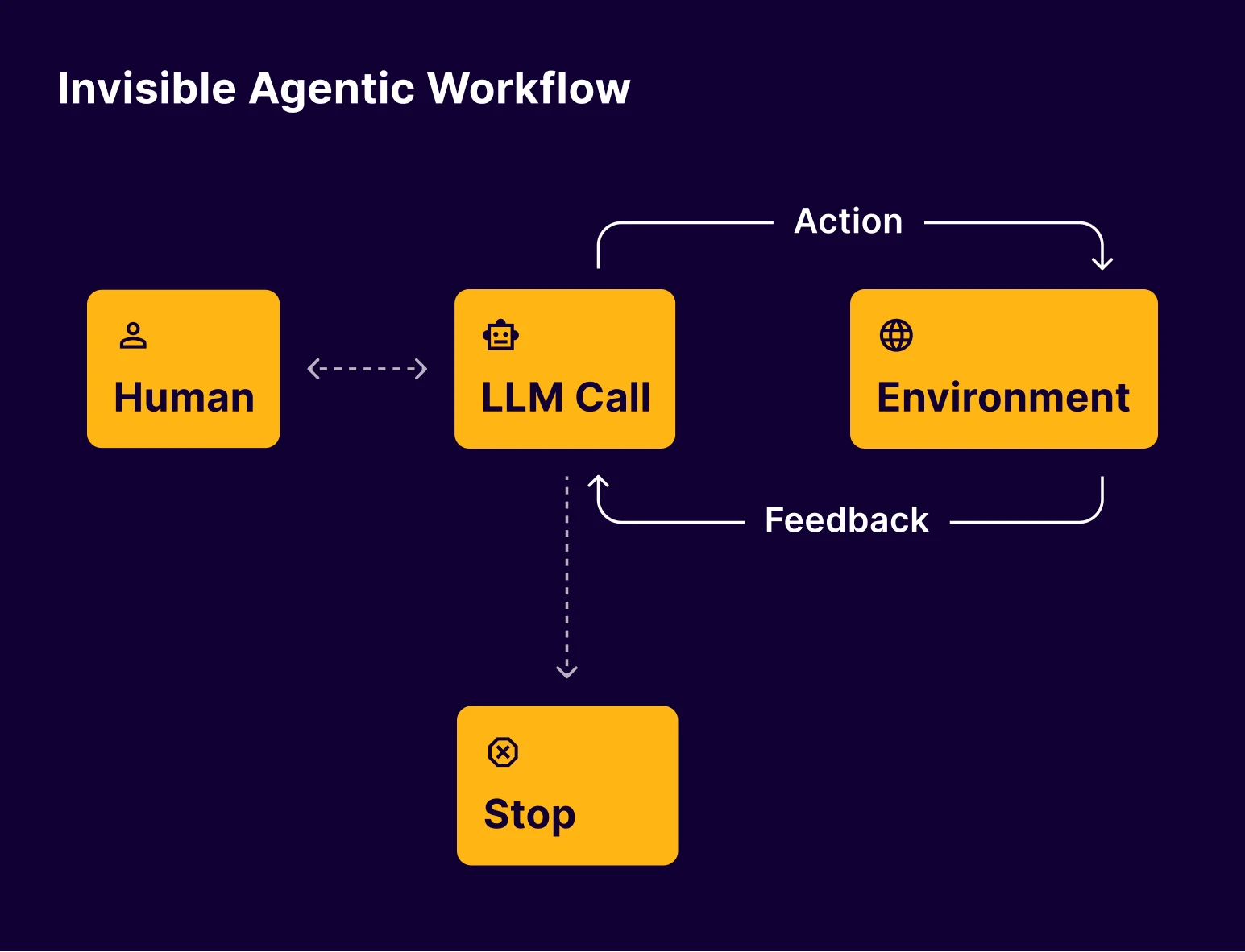
Here’s a simple breakdown of how agentic AI systems extract information, make informed decisions, and tackle complex tasks. For the sake of this example, let’s use a typical business use case of sending a follow-up email.
- A person makes a request. For example, the user enters this prompt: “Please send a follow-up email to Steve on the marketing team about my blog idea.”
- The AI agent takes action by interacting with the required environment. In our example scenario, this includes opening a browser, accessing the user’s email, and identifying the conversation referenced in the prompt.
- The agent receives feedback from the environment. For example, if the user is not already logged into their email account, the agent may be blocked from finding the email thread by the login screen.
- The agent reaches a stopping point that requires human input. To complete the original request, the user will need to log into their email account. At this stopping point, the agent will return to the user and request them to log in.
- The agent repeats steps 2-5 until the request is complete. Once the user has logged into their email, the agent will once again attempt to find the conversation referenced in the original prompt. If no further blockers come up, the agent’s stopping point will likely be to get confirmation from the user that the correct email chain has been identified. Once confirmed, the agent will draft the email within its browser and come back to the user once again to confirm that the draft is appropriate and ready to send. Finally, the agent will send the email.
Enterprise applications of agentic AI tools and solutions
In day to day life, AI agents have the potential to help us tackle some of the mundane, administrative tasks that seem to use up our free time, like ordering groceries and booking flights and hotels. With an AI assistant like OpenAI’s newly launched Operator, we can simply direct the agent to complete specific tasks, such as book a hotel room for a trip, direct it to find trip details from your email, and move on to other tasks while the assistant works in the background.
Enterprises, which typically require far more mundane and administrative tasks than an individual has in their daily life, can take advantage of AI-powered autonomous agents for a wide variety of use cases. Below are a few examples.
AI assistant use cases for sales, marketing, finance, and more
While large sales teams often have a toolbelt of applications and databases available to them, they usually have to hunt for relevant information on specific deals and accounts. While reporting capabilities provided within common sales tools can help, an AI agent can extract and surface relevant information, analyze data, and take action based on data — leaving sales workers to focus their time engaging with prospects and closing deals.
For example, a sales representative may need to follow up with a contact at a specific account a few days after a meeting. Typically, this might involve manually looking up the account, key contacts, and reviewing meeting notes in the sales CRM, finding relevant sales collateral in the content hub, and then drafting and sending an email.
With an AI agent that can tackle these complex tasks and integrate with all the aforementioned tools, however, the sales rep can simply instruct it to send a follow up email to key contacts in the account. The agent will extract relevant information and assets from the CRM and content hub, draft the email, and ask the sales rep for approval before sending it off — shaving several minutes of effort for the rep. For a sales team that sends hundreds of these communications every day, this could add up to hours of saved time.
Similar AI assistants can be deployed to serve workers in marketing, finance, and data science. Technology teams can likewise be empowered to move much faster and more efficiently with AI assistants that generate, run, and test code. Integrating such AI capabilities into business ecosystems can help enterprises across industries become more streamlined, efficient, and profitable.
AI Agents in inventory and supply chain management
Agentic AI use cases aren’t just limited to assistants. Their research and decision-making abilities combined with adaptability can also lead to powerful process automation or orchestration solutions.
Retailers and manufacturers, for example, may struggle to balance their inventory needs with supply chain availability. While the data they need is available, it can be stored in multiple databases, and reporting can be difficult to synthesize for reliable decision making.
An AI agent can go a long way toward process optimization when it:
- Integrates with these databases
- Extracts relevant data
- Sends required communications to ensure shipments and deliveries with minimal oversight from human workers

Agentic AI in healthcare
Agentic AI is also positioned to make waves in healthcare by improving both patient care and efficiency. Potential use cases include:
- A virtual medical assistant that can autonomously triage patients based on symptoms, medical history, and real-time vitals
- A hospital or clinic administration assistant that can optimize hospital workflows by dynamically adjusting staff schedules, managing inventory, and predicting equipment maintenance needs
- A drug discovery researcher that autonomously analyzes molecular interactions and simulating clinical trials
- A personalized healthcare coach that monitors chronic conditions, alerts healthcare providers when necessary, and provides real-time recommendations
Agentic AI in customer service
Agentic AI can take automated customer service to the next level by autonomously handling inquiries, resolving issues, and personalizing interactions at scale. Unlike traditional chatbots, these AI agents can adapt to customer emotions, context, and preferences, providing a more seamless experience. Potential use cases include:
- A 27/7 virtual customer support representative that can handle routine customer queries, troubleshoot technical issues, and escalate complex issues when necessary
- A personal shopping assistant that can recommend products, track orders and returns, and overall reduce friction in the buying process
- A guide for complex processes such as loan applications, helping customers get past required red tape faster and more easily.
Implementing a failsafe agentic AI strategy for enterprise
We’ve established how much transformative impact AI agents stand to drive, but big dreams and budgets aren’t enough to fuel a successful AI project. In this section, we’ll explore some of the common challenges that agentic AI projects will likely face, and first steps that AI leaders and teams can take to address them from the outset.
Common blockers and challenges to agentic AI success
Many of the biggest challenges that impede generative AI initiatives in the enterprise will likely apply tenfold to agentic AI. These include:
- Board-championed goals that prioritize launching AI rather than solving for a specific enterprise problem
- Models that struggle to meet the “last mile” of benchmarks even with large volumes of training data
- AI applications that fail after deployment due to adoption resistance in the organization
Training agentic AI models will also require specialized datasets that typical human data providers may not have the ability to deliver. While these models require just as much data as generative AI to train, gathering that data can become an additional challenge that delays projects.
The first 3 steps to a successful agentic AI initiative
Agentic AI projects can easily become a drain on budgets if they’re not carefully planned. Before kicking off an agentic AI project, be sure to do the required research and set a clear path to success.
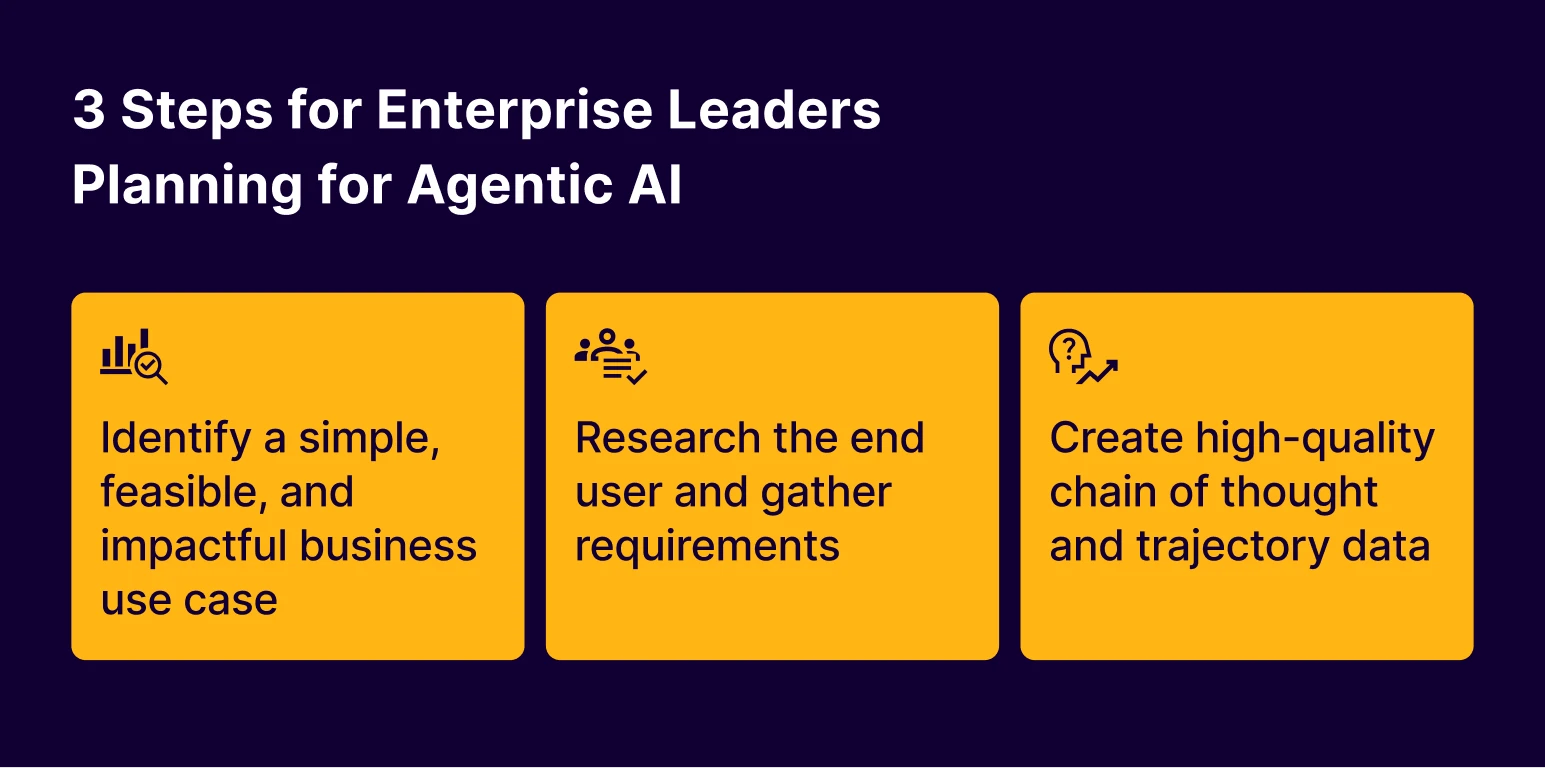
First, identify your use case
Many leaders and teams make the mistake of launching an agentic AI project for the sake of deploying AI — but successful projects begin with the problem, not the solution. Because agentic AI is still an emerging technology, choose a relatively simple use case that’s positioned to have impact (and ROI) for your pilot.
Next, research your end user
One of the most disappointing failure states for AI projects is low adoption. To prevent this, AI teams need to involve their end user from the beginning. After all, agentic AI requires human supervision, so knowing the humans who will be supervising your application and how they will prefer to interface with it is paramount to success.
Gather data on their current workflows and processes which will be transformed by the AI agent, conduct interviews or focus groups to better understand how the agent can integrate and become useful.
Gaining goodwill and buy-in from end users from the outset can both ensure adoption after deployment and give you access to their domain expertise — an invaluable asset during AI project planning and training data strategy.
Finally, create a comprehensive training data strategy
Your training data strategy should be made with input from AI leaders, researchers, and operations leaders with plenty of experience in deploying AI successfully. Sourcing high quality training data is likely to be the most time consuming and generally challenging aspect of building an AI agent. Careful planning, frequent model evaluations, and an adaptable data strategy will be key to ensuring that the model meets performance requirements without overextending the budget.
Sourcing training data for an agentic algorithm is particularly challenging, requiring specialized multi-modal chain of thought and trajectory data that’s more complex and time-consuming to generate than the prompt and response text data typically made for large language models (LLMs). Training data for agentic AI models will need to be highly tailored to the specific use case at hand and require real-time, contextual, and domain-specific data.
Glossary: General terms to know if you're new to agentic AI
Chain of thought prompting
Chain of Thought (CoT) prompting is a technique in training generative and agentic AI where models are guided to break down complex reasoning tasks into sequential, logical steps. By explicitly prompting AI to articulate intermediate reasoning, CoT improves problem-solving and decision-making processes, enhances interpretability, and reduces errors in decision-making.
This training method is particularly valuable in agentic AI applications, where autonomous systems must plan actions, adapt dynamically, and justify conclusions. CoT prompting enables more structured, human-like reasoning, making AI agents more reliable for applications like medical diagnostics, financial analysis, and multi-step customer interactions, ultimately improving transparency and trust in AI-driven decision-making.
Chain of thought reasoning
Chain of Thought (CoT) reasoning refers to an AI system’s ability to break down complex problems into logical, step-by-step processes, enhancing accuracy and interpretability. In generative and agentic AI applications, CoT reasoning measures models’ ability to navigate multi-step tasks, adapt dynamically, and make more transparent decisions.
Generative AI
Generative AI refers to artificial intelligence models that create new content, such as text, images, audio, or code, based on learned patterns from vast datasets. Unlike traditional AI, which primarily analyzes and classifies data, generative AI can produce human-like responses, generate realistic visuals, compose music, and even write software.
Generative AI models power applications like chatbots, content creation tools, and design assistants. By leveraging advanced architectures like transformers and diffusion models, generative AI enhances creativity, personalization, and automation across industries, from marketing to healthcare, making it a transformative force in digital innovation.
Machine learning
Machine learning (ML) is a subset of AI that enables computers to learn patterns from data and make predictions or decisions without explicit programming. ML models improve over time by analyzing new information, refining their outputs through training.
These models are trained using techniques such as supervised learning (using labeled data), unsupervised learning (finding patterns in unlabeled data), and reinforcement learning (learning through rewards).
ML powers applications like recommendation systems, fraud detection, speech recognition, and autonomous systems. By automating data-driven decision-making, ML enhances efficiency and innovation across industries, making it a foundational technology in modern AI development.
Trajectory data
Trajectory data refers to the sequential records of actions, decisions, and environmental states that an AI model or agent experiences over time. In generative and agentic AI applications, trajectory data is used for training models to understand cause-and-effect relationships, optimize decision-making, and improve adaptability.
Trajectory data is commonly used in reinforcement learning, where agents learn optimal strategies by analyzing past trajectories. This data enables AI models to refine responses, predict outcomes, and enhance autonomy in applications like robotics, autonomous systems, and interactive assistants.