AI holds incredible promise. But most AI initiatives are failing.
It’s a stark reality for business leaders responsible for investing in and implementing the technology in their organizations: they’re finding themselves in a frustrating cycle where their AI investments are failing to move beyond proof of concept. Because of this, executives are beginning to question ROI and their teams are scrambling to chase expensive talent and upgrade infrastructure to dig themselves out.
In 2025, it’s clear that business leaders need to reassess their approach to adopting AI from first principles. Otherwise, they risk falling into the AI Death Trap.
What is the AI Death Trap?
The AI Death Trap is when an organization mistakes AI’s long-term potential for short-term promise, focusing their priorities around AI as a magic bullet to their challenges. When AI can’t meet this impossible standard, the least prepared organizations blame the technology.
From our vantage point as a strategic partner, both the world’s leading technology companies that develop frontier AI models and the innovative enterprises that deploy those models, there are recurring patterns that indicate most organizations are, in fact, viewing AI as a magic bullet.
The patterns tend to look like these three strategic pitfalls:
- They apply AI as they would traditional software
- They misjudge the real progress they are making, reaching 80% fast but struggling to close out the last mile
- They fail to recognize and build systems around AI’s strengths and weaknesses
The result? Anywhere from 70-90% of AI POCs don’t go into production.
But while the AI Death Trap reveals the misconceptions organizations have about artificial intelligence, the root causes of AI pilot failures run even deeper. Understanding these fundamental challenges is crucial for any organization looking to move beyond superficial AI implementation.
5 Reasons AI Pilots Fail
1. A lack of a success criteria
Ironically, this is the most common problem and also the easiest to address. Most AI initiatives die on the vine simply because leadership teams haven’t defined what success looks like when it comes to their experimental R&D projects. Can you articulate exactly how AI will improve productivity, reduce costs, or create new value? If not, you're setting yourself up for disappointment.
2. A lack of expertise and understanding
While some organizations have advanced AI/ML teams, most don’t, and hiring experienced talent is expensive and challenging. This means many are left trying to repurpose or upskill their existing teams to meet the expertise needed to build, fine-tune, evaluate, and monitor their AI. In most cases, it doesn’t work.
3. Poor data quality and availability
High quality models need high-quality data, but many organizations fall into the trap of developing AI initiatives on generic or sample data. As they attempt to move these projects into production, they hit a wall: their data is fragmented, inconsistent, or inaccessible.
4. The high cost of scaling frontier LLMs
Most organizations look to frontier LLMs for small-scale POCs. This presents two problems: They don’t anticipate how costs would scale, particularly inference costs. As the POCs enter production, the cost of inference is prohibitive. The large frontier models also become overkill for their needs. In fact, their lack of specialization can prevent them from succeeding in their role.
5. A lack of trust and compliance concerns
AI is a trust challenge as much as it is a technological challenge. Your stakeholders—from employees to customers to regulators—need transparent, accountable, and accurate AI systems. In many cases, frontier models continue to hallucinate and fail unpredictably, risking trust in your business.
A More Holistic Approach to AI
To avoid the AI Death Trap, business leaders need to take this opportunity to re-architect their organizations around leveraging the strengths of AI and humans, simultaneously, while compensating for their weaknesses.
So, what does this architecture look like?
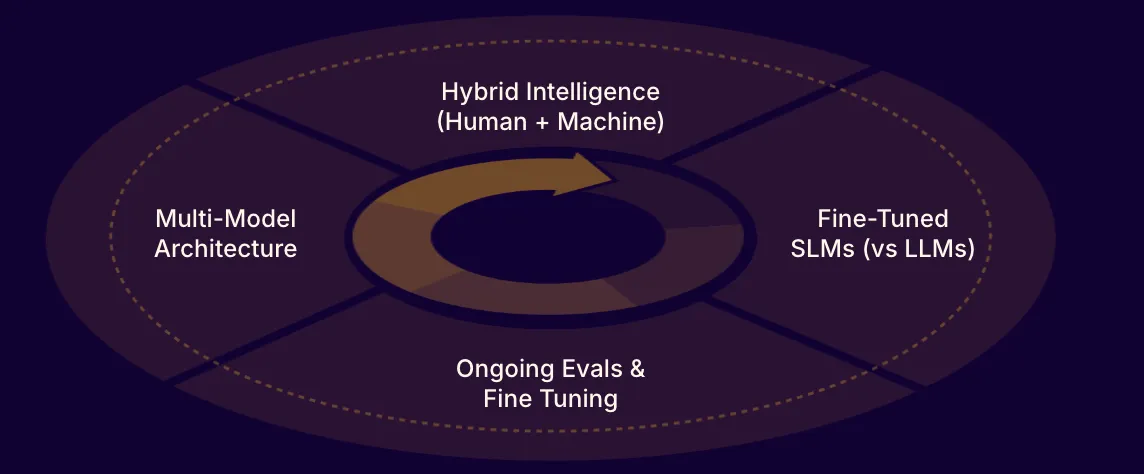
Fine-tuned SLMs vs. LLMs
For most enterprise use cases, purpose-built models, fine-tuned for that use case, will outperform LLMs at a significantly lower cost. For instance, in the financial services sector, fraud detection would require a model tailored specifically to identify nuanced patterns in transaction data, whereas a general-purpose model would risk false positives. Focusing on building the right tool for the job is what can transform AI from a promising experiment into a powerful business driver.
Hybrid Intelligence
Humans remain crucial in AI workflows. They provide oversight and alignment, and build trust. In areas like customer engagement or fraud detection, AI models can process large amounts of data and make predictions, but human oversight helps verify the accuracy and relevance of these predictions. Systematizing human involvement at every layer ensures that AI outputs are effective by maximizing AI’s strengths and minimizing its weaknesses.
Adaptive, multi-model infrastructure
Modern, flexible infrastructure—built on modular architectures—is crucial for making AI a consistent part of business operations, rather than just an isolated project. What does that infrastructure enable? Operating multiple models for different use cases and chaining multiple models in the same use case or workflow while properly integrating human input along the way.
A commitment to continuous learning
AI systems must be treated as dynamic, living components of an organization. The most successful AI initiatives continuously refine and retrain models, adapting to new data and evolving business needs. The tighter you can integrate this feedback loop, the faster your models will improve, and the more confidence you will gain.
Why an AI Partner is Your Ticket to Success
The good news: it’s not too late to start, but you shouldn’t try to start it alone. With AI experience expensive and in short supply, adapting to the rapid pace of AI evolution is nearly impossible to keep up with.
The winning businesses of 2025 and beyond will leverage the expertise and experience of others, like Invisible, who are at the forefront of AI model development, understand your challenges, and align with your goals.
Want to escape the AI Death Trap? Learn how Invisible can help your business move beyond POC and unlock real value.