Around 80% of artificial intelligence (AI) initiatives are doomed to failure, according to recent research. While some projects fail due to the lack of quality data or expertise in either AI engineering or relevant domains, a primary cause of failure is the lack of clear success criteria. Many enterprise AI or R&D teams become stuck in the experimentation and proof of concept (POC) stages of projects without a clear path forward, often using up budgets without providing ROI. These projects become fairly typical AI failures.
In this blog post, we’ll explore how AI and innovation leaders can prevent these AI failure scenarios by establishing clear success criteria supported by data and research.
Want to learn more about how you can launch better artificial intelligence projects that drive ROI and adoption at your organization? Learn how you can avoid the “AI Death Trap” of failed projects.
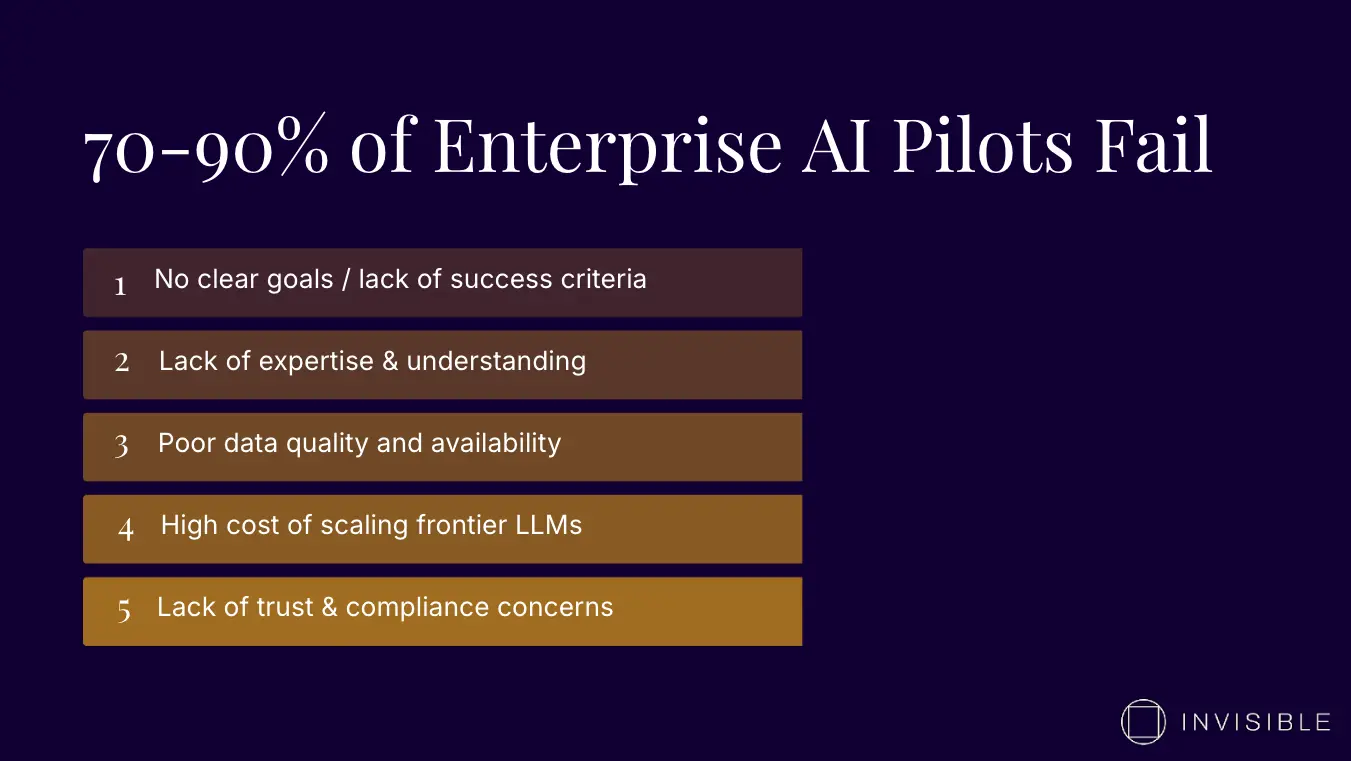
Step 1: choose the right problem to solve with AI
Many leaders are feeling the pressure to launch AI solutions from their boards and markets, making it easy to get drawn into an AI-first approach when choosing your projects. While an agentic application built on one of the world’s leading large language models may sound impressive, establishing this as the end goal is the most common way that leaders put the cart before the horse.
Your first step in building your AI project strategy is to start with the problem rather than the solution. Most organizations have plenty of problems that can be addressed with AI or machine learning — there’s almost always a process that can move faster or become more accurate, a manual task that needs to be scaled, or information that can be more easily accessed and made digestible.
To choose the right problem to address with your AI project, take the following into consideration:
- AI solutions take time. The problem you solve should therefore be unlikely to change for several months.
- Solving for the issue should provide enough value to the business. Choose an issue that needs to be solved, not a “nice to have.”
- Understand that AI can’t solve everything — yet. Consult with AI, domain, and other technical experts to choose a problem that can reasonably be solved with an AI application.
Once you’ve chosen a specific problem to address with an AI application, you can start laying out your success criteria. If the problem is a slow or heavily manual process, for example, you can set a goal for how much time should be saved on the process with the use of your AI application.
Step 2: research your end user
While identifying a problem is important, the adoption and implementation of your AI application depends entirely on the people who are going to be using it in their day to day work. That’s why researching your end user audience via focus groups or individual interviews is key to launching a successful AI application in the real world.
While the questions you need to ask your audience depends on the use case and end user, here are some questions that can help guide your research:
- The current situation. What does the average end user’s current workflow look like, and how would they like it to be different? What workarounds or “hacks” have they implemented to solve for the problem at hand?
- How do they receive information? If the AI application will likely deliver information to the end user, what’s the most helpful format and context for that information to become available?
- Technical aptitude. What average level of technical expertise does the end user have? How much upskilling and training will they need for an AI application to be useful? How can your AI developers meet the end user in the middle in terms of technical aptitude and reduce friction for adoption?
- Adoption blockers. What are the end users’ attitudes towards AI? Are they willing to use an AI-powered application in their regular work? What hesitations or concerns do they have about using generative AI?
End user research can help to establish more clear success criteria for your AI project, as well as establish rapport between them and the AI development team. This can make the experimentation and adoption stages of your AI project much easier and faster.
Organizations that include end user research as a part of a holistic AI model evaluation strategy are much better positioned to launch successful AI applications that deliver ROI.
Step 3: build your hypothesis
Once you have a clear understanding of the problem you’re solving and the people your project will affect the most, your team will be much better prepared to propose potential AI tools or solutions.
At this stage, you can propose specific AI applications tailored to your problem and audience. These hypotheses should enable end user productivity and creativity while reducing time spent on repetitive, manual, administrative tasks.
Other elements to consider when building your proposal include:
- Building vs. adapting. Building a large model from scratch takes the most investment from a time and cost perspective, but it might be necessary for your use case.
- Evaluating models on specific tasks vs. general performance. Generic leaderboards can be a starting point for choosing a model to adopt for your use case, but evaluating them for your specific use case is key to making a fully informed decision.
- Consider all options, not just the popular ones. Small language models may not be making as many waves as LLMs or agentic AI in the public imagination, but they might be a much better choice for your use case.
Your hypothesis should also include research on the costs and value of the application, along with how much and when the business can expect to see ROI. Gartner’s model for calculating costs and value can be a useful place to start.
At this point, your goals and success criteria should be clear, taking into account both the specifics of your business problem and the end users that it will most significantly impact.
Want to learn more about how you can launch better AI projects that drive ROI and adoption at your organization? Learn how you can avoid the “AI Death Trap” of failed projects.